State lawmakers face difficult choices about how to use scarce public resources. To ensure that investments have a big impact on young children, lawmakers often want to know—which policies actually work?
The Prenatal-to-3 Policy Impact Center at Vanderbilt University can answer that question. To identify effective policies, we read thousands of research studies, and then we narrow the list down to only strong causal studies with well-executed research design and rigorous analytic methods.
For the Center to label a policy or program effective, it must have been studied through rigorous research, defined by being able to establish a causal link between a policy or program and an outcome. We rely on two types of study designs to identify causal relationships in policy evaluation: randomized control trials (RCTs) and quasi-experimental designs (QEDs). Our standards are high, but necessary to guarantee the best policy recommendations for children and families.
Making Causal Connections
If a state implements a new policy (e.g., Medicaid expansion) and infant birth outcomes improve, did birth outcomes improve because of the policy change? Or because of something else that happened to coincide with the policy change, for example the introduction of a new medical technique or drug? Even an expert observer can’t tell just by watching the situation unfold. And not all research studies can answer the question.
Rigorous research can tell us whether a policy or program directly caused the improvement, isolating the impacts of a policy on specific outcomes. A causal relationship is the key part of a rigorous study and allows us to say with confidence that a policy or program is likely to help, hinder, or have no effect on an outcome. So, what does a causal relationship look like in research?
Randomized Controlled Trials
The gold standard for establishing causality is an RCT, in which participants are randomly assigned to one of two study groups:
- the experimental group, who receive the new intervention, and
- the control group, who receive standard practice.
For example, from a population of interest, a researcher can randomly assign a group of pregnant people who are interested in trying a new prenatal care approach to either participate in group prenatal care or not. To understand the program’s impact on outcomes of interest, the researcher can compare the experimental group’s outcomes to those of the group of people randomly assigned to the control condition, or “typical” care. In this case, the control group most likely receives individual prenatal care.
RCTs are the gold standard in research because they eliminate what is known as selection bias. Selection bias refers to the situation in which people who choose to participate in a new program or policy may differ from people who do not choose to participate. It is the differences in characteristics (e.g., motivation, knowledge, access, or privilege) between those who choose to participate and those who do not that lead to differences in outcomes, rather than the intervention itself.
In the example of group prenatal care, random assignment among those who are interested in participating in the program is key. If pregnant people who enjoy being around other people and who have no serious health concerns about their pregnancy are more likely to choose to participate in group prenatal care, then without random assignment to the program or care as usual, a researcher may attribute better health and social outcomes to the program rather than to the pregnant people who chose to participate in the first place.
And yet, RCTs are typically not possible in policy evaluation, due to cost considerations, difficulty randomizing, small sample sizes, and ethical concerns. Typically, RCTs are more common in evaluations of programs rather than policy interventions. Policy interventions happen on a larger scale, across an entire state or country, and creating random assignment is usually impossible. Programs happen at a smaller population scale and have more opportunities for random assignment.
Quasi-Experimental Designs
Like RCTs, QEDs help make causal connections. But instead of randomly assigning study participants to an experimental or control group, QEDs take advantage of naturally occurring variation to identify impacts. One natural source of variation is the implementation of a policy in one state and not another. A QED’s strength depends on how well the researcher reduces or eliminates selection bias and improves validity of the comparison group.
For example, through Family Connects, hospitals offer families a postpartum home visit by a specially trained nurse, who connects the family with community resources. A hospital may only offer Family Connects for births occurring on weekdays. This system would create a natural experimental group of patients seen on weekdays and a natural comparison group of patients seen on weekends. If researchers assume the only difference between families in each group is the timing of their births (and no external factors may influence this), researchers can conclude that any differences in outcomes arise from the Family Connects program.
Another common method for examining policy interventions in large populations is a differences-in-differences (DID) approach. In this method, researchers compare two similar groups before and after real-life implementation of a policy. For example, when a state implements Medicaid expansion, a DID approach can be used to compare outcomes in two states—one that implemented Medicaid expansion and one that did not. The study compares differences between outcomes before and after the time of Medicaid expansion in each state (the differences between the differences, hence the name).
In this case, the non-expansion state serves as the control group, reflecting the outcomes that would have occurred if Medicaid expansion were never enacted in the experimental group (the expansion state). A well-executed DID design leverages the fact that the primary difference between the groups is whether they live in a location experiencing the policy intervention.
Limitations of Causal Research
At the Center, we recognize the shortcomings common in policy research. Historically, research has exacerbated racism and inequalities and has not taken all groups of people into consideration. For example, the fact that a program or policy works for a White family with a higher income does not mean there will be similar effects for a Black family with lower income.
Additionally, rigorous causal studies can be expensive and require a large sample. These requirements often leave smaller, newer programs without rigorous evidence to support their positive outcomes. Finally, even the most rigorous research will have shortcomings. Generalizability can be limited, and the replication of results in a different setting or sample can never be certain.
For all these reasons, we recognize the other types of research that inform policymaking. Although our evidence reviews focus on causal research, we acknowledge the value of non-causal research. Qualitative research, for example, can provide more detailed context on policy changes. These details include a deeper understanding of impacts—including unintended consequences, implementation challenges, and additional insight on outcomes of interest or outcomes difficult to measure—and why impacts may have occurred.
Rigorous Research is the Foundation of What We Do
We primarily rely on rigorous RCTs and QEDs to identify causal links between policies and beneficial outcomes for children and families. These types of studies allow us to identify and share effective policies. Using this information, we strive to inform and accelerate evidence-based policymaking in every state.
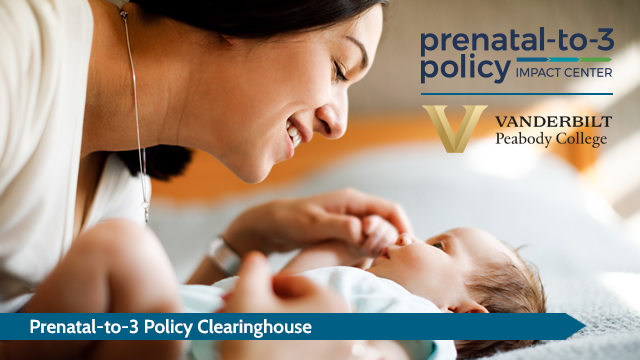